There’s nothing new in using analytics in data protection or breach prevention. Firewalls, for example, analyze packet contents and other metadata, such as IP addresses, to detect and block attackers from gaining entry. And anti-virus software is constantly scanning file systems for malware by looking for bits of code and other signs that a file is infected.
Unlike firewalls and anti-virus software, User Behavior Analytics or UBA focuses on what the user is doing: apps launched, network activity, and, most critically files accessed (when the file or email was touched, who touched it, what was done with it and how frequently).
Get the Free Pentesting Active
Directory Environments E-Book
UBA technology searches for patterns of usage that indicate unusual or anomalous behavior — regardless of whether the activities are coming from a hacker, insider, or even malware or other processes. While UBA won’t prevent hackers or insiders from getting into your system, it can quickly spot their work and minimize damage.
Yes, UBA is a close cousin of SIEM (Security and Information Event Management). SIEM has traditionally focused on analyzing events captured in firewalls, OS, and other system logs in order to spot interesting correlations, usually through pre-defined rules. For example, several login failure events in one log might be matched to increased traffic exiting the network recorded in yet another log. SIEM might decide this is a sign of hackers entering the system and removing data.
As we’ll soon see, by focusing on perimeter systems and OS logs instead of the data itself, it’s easy to miss insiders abusing their access, as well as hacker activity, because the hackers have become very good at appearing like ordinary users once they’re inside. That’s where UBA comes in. By focusing less on system events, and more on specific user activities, UBA can learn user patterns and then zero in on hackers when their behaviors differ from legitimate users.
For those who want a more traditional definition of UBA, research firm Gartner has come up with the following:
User Behavior Analytics (UBA) [is] where the sources are variable (often logs feature prominently, of course), but the analysis is focused on users, user accounts, user identities — and not on, say, IP addresses or hosts. Some form of SIEM and DLP post-processing where the primary source data is SIEM and/or DLP outputs and enhanced user identity data as well as algorithms characterize these tools. So, these tools may collect logs and context data themselves or from a SIEM and utilize various analytic algorithms to create new insight from that data.
So Why UBA?
Great question. To understand why UBA came about, you have to consider where current data security approaches have fallen short. For anyone who’s been following the headline-making breaches over the last two years, it’s almost as if the hackers had been given the keys to the front door.
In the case of Snowden or WikiLeaks, the hackers may literally have had the keys to the front door, as they were already inside. In the Target breach the hackers either obtained or guessed a password of a remote login. In the recent Office of Personnel Management incident, the attackers tricked an employee into downloading malware through a phishing attack.
Defending the inside from legitimate users is just not part of the equation for perimeter-based security, and hackers are easily able to go around the perimeter and get inside. They entered through legitimate public ports (email, web, login) and then gained access as users.
Once in, hackers have become clever at using malware that isn’t spotted by anti-virus software. Sometimes they even use legitimate sys admin tools to conduct their cyber work.
In fact, to an IT admin who is just monitoring their system activity — by examining apps used, login names, etc. — the attackers appear as just another user.
And that’s why you need UBA!
Perimeter-oriented security technology is looking for unusual activity in the wrong places. The new generation of hackers are just not tipping their hands by accessing unauthorized network ports or through software with known signatures.
Undetectable: Malware and Attack Vectors That Go Around Perimeter Defense
We know the hackers involved in the big retails breaches were good at stealthily entering and leaving the data center.
But how were they actually getting away with it?
The techniques of the hackerrati follow two steps: entering through public access points and then inserting specially crafted malware that flies below the radar. This combination has proved to be incredibly effective.
The low-level details of the hack craft can be quite complicated, and it’s something we’ll cover in future posts. In the meantime, here’s a brief overview of their techniques.
Entering Undetected: Bad Passwords, Phishing, and SQL Injection
Password guessing, unfortunately, is still a very effective way for hackers to enter systems. It works because users all too often choose easy passwords — some variation of their name or simple numeric sequences — or even leave the default passwords as is for installed software or firmware (databases, routers, etc.).
Consider successful password guessing the cyber equivalent of picking a low-tech door lock.
In recent years, however, hackers have become very good at getting employees to invite them in by simply sending an email—no password guessing required. This attack vector is known as phishing.
Think of phishing as a way to disguise an email, known as a phish mail, so it appears to be sent from a legitimate source—say an over-night shipping service. Hackers will also re-use official corporate logos in the email’s contents to make the phish mail even more convincing.
Hackers count on the fact that the average corporate user is not technically knowledgeable enough to understand the underlying structure of URLs. So it’s easy for them to make believable forgeries of the sender’s email address.
For example, many average users would likely accept that jon@fed3x.com is an employee at FedEx. Why? The address looks enough like the legitimate domain, fedex.com.
Once lured, the employees click on a link or attachment, which launches the malware. Mission accomplished: they’re now inside.
A third way for hackers to gain entry is through an SQL injection attack. It’s similar to phishing in that entry is through a public access point — in this case, a web site.
In SQL injection, attackers take advantage of bad web application code that fails to sanitize user input.
Here’s how this happens. When a user enters text into a web form, it often triggers a request to an SQL server to pull up a relevant database record. However, if the input string as is not properly sanitized, it’s possible for the hackers to insert evil SQL code, which is then sent as is to server. The evil code gives them a foothold, allowing hackers to launch a shell or access basic OS commands.
Stealthy Stealing: APTs and Command and Control (C2)
Once in, the next item on the hacker’s to do list is to install malware that supports basic administrative capabilities: at a minimum, file upload or download, simple commands, and searching directories. Known as RATs (remote administration tool) or more broadly as Command and Control (C2), the malware can be accessed by the hackers from their own domains.
This is not a new idea: it’s as old as the first botnets. The real innovation by hackers has been the combination of C2 malware and overall stealthiness.
The result is Advanced Persistent Threats or APTs.
By the way, a C2-style APT was implicated in the giant Anthem breach – the Derusbi APT.
How do these APTs work?
The hacker inserts the RAT logic into Windows can’t-live-without-‘em system DLLs, where they remain hidden. Once the DLL is activated, the hackers then can send commands and receiving results.
In addition, the RAT’s can communicate with a central control server — typically within its own legitimately registered domain—over standard HTTP or HTTPs. They can even connect with a fake DNS server, which is expecting RAT commands hidden in the special DNS protocols — that was the case with Anthem.
There you have it. Malware that’s embedded in standard Window’s software and communicates over vanilla Web connections. This is very, very hard to detect and block.
Hacking Breach Stats
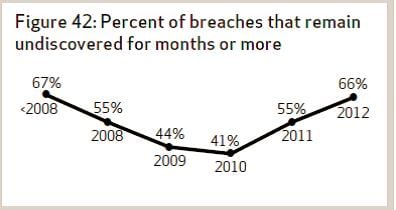
The Verizon Data Breach Investigation Report (DBIR) is a great source of statistics on hacking. One statistic they’ve been tracking over the last few years is the time it takes for organizations to discover they’ve been breached.
The first bit of bad news is that the unit of measure is months. The next is that the trend has been getting worse, not better.
In 2012, almost 70% of the breaches in Verizon’s sample took months for the organizations to discover.
Speaks volumes about the stealthiness of the hackers using some of the techniques we discussed above.
Flavors of UBA
Gartner broadly divides the UBA software into two categories: those that rely on “canned” analytic rules to spot abnormal behavior, and those that base their analytics on dynamic or customized models.
For example, in a canned rule, an administrator might decide to force a notification if a sensitive file is accessed on the weekends between 12 and 5 AM.
In pure dynamic rule making, the underlying UBA engine decides what’s normal (see below) and detects activities that fall outside this normal range. In other words, the engine create its own internal rules.
Obviously, there’s a place for both canned and dynamic rulemaking in UBA. But keep in mind, UBA software relying solely on canned rules would then require an IT security administrator with great instincts on what hackers are up to. Not many administrators have those wizard-like powers.
Another dimension in UBA software is the source used for the underlying data. Some UBA solutions focus primarily on network and perimeter system activity (logins, apps, events). Other UBA-variants will focus on more granular metadata in the system itself—like user activity on files and emails.
The drawback with a pure network or system-based approach, as I’ve pointed out above, is that it’s hard to detect hackers who’ve entered by email or injection and then stolen the credentials of existing users, unless you’ve got UBA intelligence. At a high level, their logon and app usage will not appear out of the ordinary.
UBA variants that look at user activity on files and emails will have a far better chance of spotting this type of attack. The key is, at some point, the hacker-posing-as-legitimate-user will attempt to search for and copy files containing sensitive data—that’s where the gold is.
What’s Normal?
UBA is based on the idea that by knowing what users on a system are doing — their activities and file access patterns. Ultimately, the software has to derive a profile that describes what it means to be that user. So when a hacker steals the user’s credentials and accesses data he rarely visits, his activities will now differ from the profile.
For the whole thing to work, UBA has to have a track record of the user — an average or measure of normal behavior. You can say the UBA software has to be trained to identify normal behavior — typically involving processing the actual activity logs –file access, logins, network activity — over an extended period of time.
If you’re thinking that some of the classification and prediction techniques of Big Data analysis – nearest neighbor, regressions, Bayesian—are appropriate for UBA, you’d be right. But whatever the exact method used, the analytics will establish a baseline from which it will be possible to predict what’s normal and what’s not.
What to Look for in UBA
Not all UBA software is the same. As I suggested earlier, UBA that supports only canned rules and pure perimeter-based analysis won’t be able to keep pace with clever hackers. UBA that has access to granular file and email activity has a better shot. Remember: files and emails are often what cyber thieves are after, and at some point they’ll make run for that data.
Here’s a list of essential features for UBA software that can take on the new generation of hackers:
- Process vast amounts of user file and email activity. File systems are enormous and sensitive data can be spread out like the proverbial needle in the haystack. To spot the hackers, the UBA engine should be able to search through and analyze the key metadata and activity of many users across huge volumes of data.
- Determine a baseline of “normal” file and email access activities. You’ll need historical data about your employees’ activities. The UBA engine therefore should have intimate knowledge of file metadata—access times, users, permissions, etc. Only with granular, event-level file and email activity can the UBA’s underlying prediction and machine learning software produce accurate profiles of average user behavior. It can then accurately decide whether a hacker or insider has taken over an employee’s account.
- Real Time Alerts. The UBA software must be able to track file activities across a large user population in real-time. Its hacker-detection algorithms must also make decisions in near real-time — not just at the end of the day. The time window for when they touch and copy the sensitive data can be small. So the UBA software has to be ready to react quickly.
References
Breaches
http://www.threatconnect.com/news/the-anthem-hack-all-roads-lead-to-china/
http://krebsonsecurity.com/2014/01/new-clues-in-the-target-breach/
http://www.verizonenterprise.com/DBIR/2014/reports/rp_dbir-2014-executive-summary_en_xg.pdfPhishing
https://itunes.apple.com/us/book/anatomy-of-a-phish/id824386033
Malware, APTs, C2
http://intelreport.mandiant.com/Mandiant_APT1_Report.pdf
http://www.akamai.com/dl/akamai/akamai-security-advisory-web-shells.pdf
http://www.sans.org/reading-room/whitepapers/testing/pass-the-hash-attacks-tools-mitigation-33283
https://www.varonis.com/blog/closer-look-pass-hash-part-iii-ntlm-will-get-hacked/
http://www.contextis.com/resources/blog/malware-analysis-dark-comet-rat/
SQL Injection
https://www.varonis.com/blog/top-five-dangerous-software-errors/
http://pentestmonkey.net/cheat-sheet/sql-injection/mysql-sql-injection-cheat-sheet
Pass the Hash
https://www.youtube.com/watch?v=1YYtTg8sjuY
https://www.varonis.com/blog/the-definitive-guide-to-cryptographic-hash-functions-part-1/
https://www.varonis.com/blog/closer-look-pass-hash-part-one/
Predictive Analytics
http://machinelearningmastery.com/a-tour-of-machine-learning-algorithms/
SIEM, UBA
What should I do now?
Below are three ways you can continue your journey to reduce data risk at your company:
Schedule a demo with us to see Varonis in action. We'll personalize the session to your org's data security needs and answer any questions.
See a sample of our Data Risk Assessment and learn the risks that could be lingering in your environment. Varonis' DRA is completely free and offers a clear path to automated remediation.
Follow us on LinkedIn, YouTube, and X (Twitter) for bite-sized insights on all things data security, including DSPM, threat detection, AI security, and more.
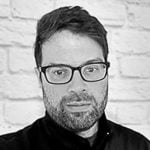