The adoption of generative AI and the rising threat of shadow AI applications has increased the risk of data exposure and larger attack surfaces. As users and organizations integrate these technologies into their operational workflows, vulnerabilities inherent in traditional data security measures become more pronounced.
Gartner’s report "Predicts 2025: Managing New Data Exposure Vectors" emphasizes the need for innovative approaches to data security, particularly highlighting the crucial role of next-generation Data Loss Prevention (DLP) solutions.
The report paints a picture without explicitly stating it: DLP needs a zero-trust strategy for the future — a Zero-Trust renaissance.
CISOs will start to prioritize "DLP products that incorporate both behavioral and intent analytics alongside content-centric analytics” to continuously trust-but-verify all data in use and data in transit.
Continue reading and watch the video above to learn more.
Challenges with traditional DLP
Traditional DLP solutions often focus solely on export threats in a ‘whack-a-mole’ fashion, triggering on known data flows and managing new ones as they pop up. Endpoint DLP solutions first hit the market in the early 2000s to prevent specific data from flowing from a device like a laptop to external drives, network-connected devices, printers, and eventually third-party apps on the browser.
In the current era, DLP solutions run in the cloud and prevent cloud-to-cloud and app-to-app data flows on top of resources like Microsoft 365, Dropbox, Slack, Box, Google Workspace, Monday, Salesforce, Workday, Zoom, and more.
And still, the list of apps used by enterprise organizations grows by the day. In fact, through AI-powered developer tools, an untrained user can spin up a cloud storage app in minutes with natural language prompting. This newly created app will not be found in any DLP list.
Managing a manually updated list of restricted apps, unallowed browsers, whitelisted domains, etc., can be arduous. Yet, creating DLP policies for the infinite export scenarios can be equivalent to a full year’s worth of effort for one to three full-time employees, given the size and scope of the business.
Below are only a few variables that exponentially compound the DLP policy creation conundrum:
- Policies for supported and non-supported file types
- Policies for managed and unmanaged/unenrolled devices
- Policies for hundreds of managed apps in the org and thousands of unmanaged apps
- Policies for risky users vs non-risky users and additional policies defining ‘risky’
- Policies for each sensitive information type
- Policies for each tag or label
- Policies for each relevant regulation or framework
You can create policies that overlap some of these areas, but generally, security and risk teams need to create dozens of policies. Each policy is a ‘trust’ statement: “We trust that any action or data flow that falls into this scenario is legitimate.”
What if we applied a Zero-Trust approach to data flows and data in use or in transit? Verify explicitly.
A zero-trust approach for data flows, whether the data is in use or in transit, would require explicit verification
The Zero-Trust approach: A paradigm shift
For a bit of context, “zero-trust” is a cybersecurity model that operates on the principle of "never trust, always verify." It assumes that threats can come from both inside the network and known entities, thereby necessitating continuous verification of user identities and granting only just enough access.
At the turn of the 21st century, new technologies developed around MFA and condition-based access. These measures and others would assume that an identity could be compromised and verify multiple points about an access request or session. If the human identity is verified by a password and then verified by a second factor like a one-time code sent to the person’s mobile device, access would be granted.
Yet Zero-Trust principles recommend that the session be on a verified device that is managed and monitored. Even after that, several more factors would continue to be monitored and checked periodically because the assumption of zero-trust is breached. Assume breach.
In traditional security models, a trusted user who is authenticated and authorized to access and share data might still pose risks if their identity and device are compromised. After all, attackers are still finding ways to circumnavigate MFA and other access checkpoints today.
What if security and risk teams applied the same zero-trust rigor to data flows as they do to identity and access to data?
Applying Zero-Trust to DLP
Continuous monitoring of new data flows
As users start to use new AI applications or cloud sources, these additional egress data flows often fall out of scope for existing DLP policies. Data can come from anywhere, as shown in the image below. Organizations need visibility into these export events and treat them as breach events.
Data flows into AI from multiple locations. Each ChatGPT conversation can be an exfiltration event.
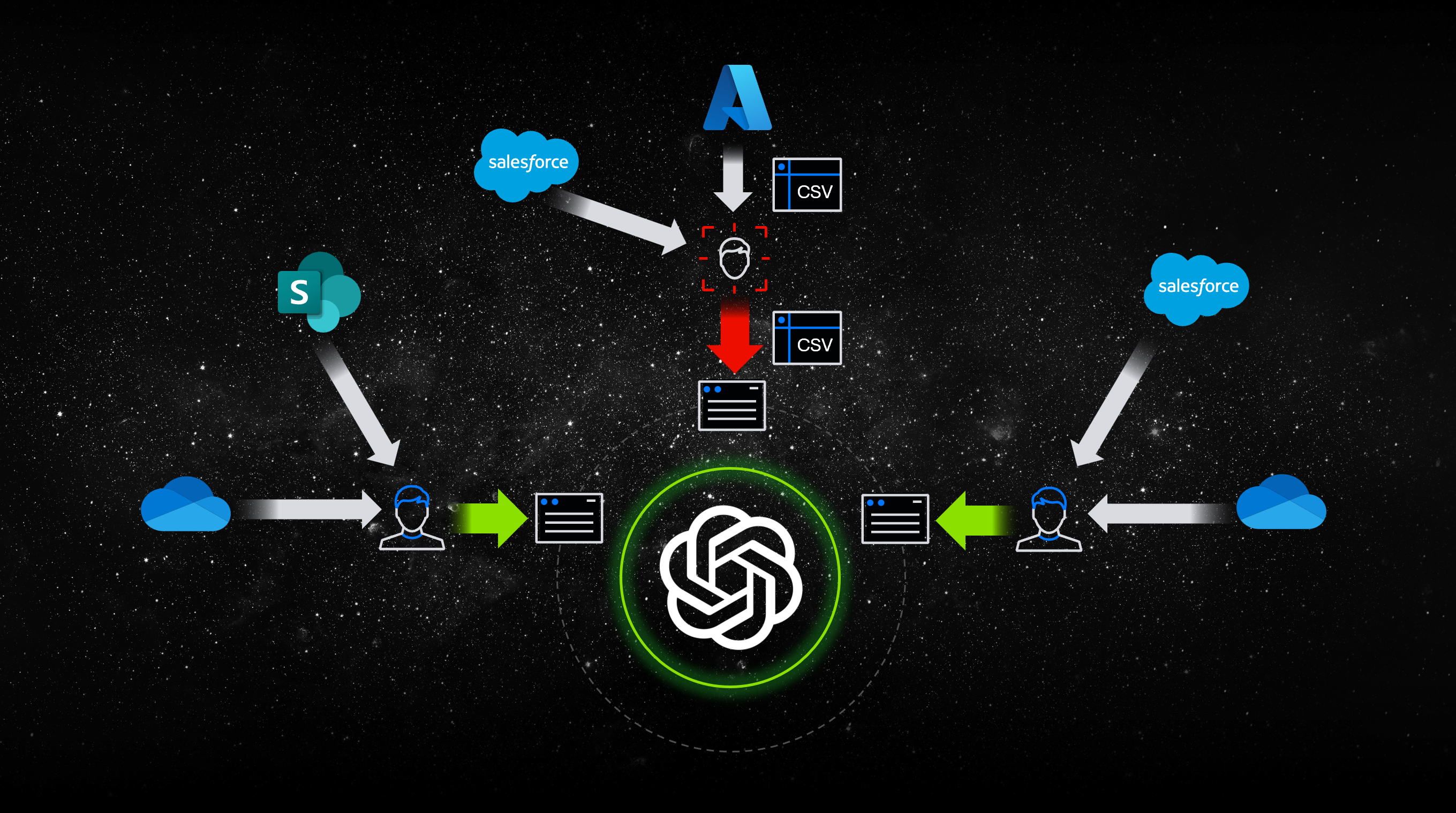
Data flows into AI from multiple locations. Each ChatGPT conversation can be an exfiltration event.
A Zero-Trust approach would require full inspection of these events to determine what sensitive information types were present in the data transmitted, context about the session (who, what device, from where, etc.), and information about the end destination.
Contextual insights can then drive truly adaptive DLP, whereby an organization can manually or automatically modify policies to fit the new vector or restrict a third-party app. Teams may also take other actions on the user or device – restrict access to a web app, block a domain for browsers, disable a user, or terminate a session.
Assuming breach and adapting to new flows will become critical as generative AI applications introduce new export scenarios that can be exploited by malicious actors or misguided internal users. These data flows, often unstructured, require robust surveillance mechanisms to detect and mitigate potential threats. DLP solutions should incorporate intent detection and real-time remediation capabilities to effectively find risks.
Detecting risky activity in existing flows
Just because a known egress vector is included in policies doesn't mean all data passing through it can be trusted. Next-generation DLP solutions need to integrate advanced user behavior analytics (UBA) and machine learning algorithms to continuously assess user activities. This ensures that even trusted users and data flows are scrutinized for signs of compromised identities, malicious intent, or improper use.
Anomalous activity scenario: Some DLP solutions on the market can adapt a user’s access based on ‘risk’. ‘Risk for these solutions is often defined by identity events like failed MFA attempts or impossible travel scenarios. Yet, malicious actors may exhibit behavior that is not seen as risky at face value, like simply downloading or sharing files.
Hackers are known to create complicated data flow paths to go unnoticed, like the example below:
- Step 1: Download files locally
- Step 2: Upload files to a ‘trusted’ third-party app
- Step 3: Use a native AI agent in a third-party app to summarize or create a new master file based on the original files
- Step 4: Upload AI-generated unmarked file to private cloud storage (owned by hacking group) or share with a hacking group directly through personal email
Noticing the frequency of download events and characteristics of files being downloaded (‘content contains ____’) and adapting policies can stop a breach before it ever reaches Step 2 again.
Malicious activity scenario: In a well-documented case, Microsoft found that attackers were compromising an identity within a trusted supply chain vendor, hosting a malicious file in SharePoint/Dropbox, and then sharing the file with known entities with a share link. After detecting this type of behavior, a policy may need to be created or modified to flag users who are creating many ‘sharing links’ in mass. Alternatively, automated DLP solutions could accommodate new and novel scenarios where a user is sending a particular file extension, such as a PDF with a certain name.
Advanced DLP solutions must employ AI-powered analytics to identify unusual patterns of data access or usage that may indicate malicious activity. By focusing on user intent and behavior, these solutions can proactively detect and respond to threats before significant damage occurs.
Even trusted data flows can become exfiltration paths if users are compromised.
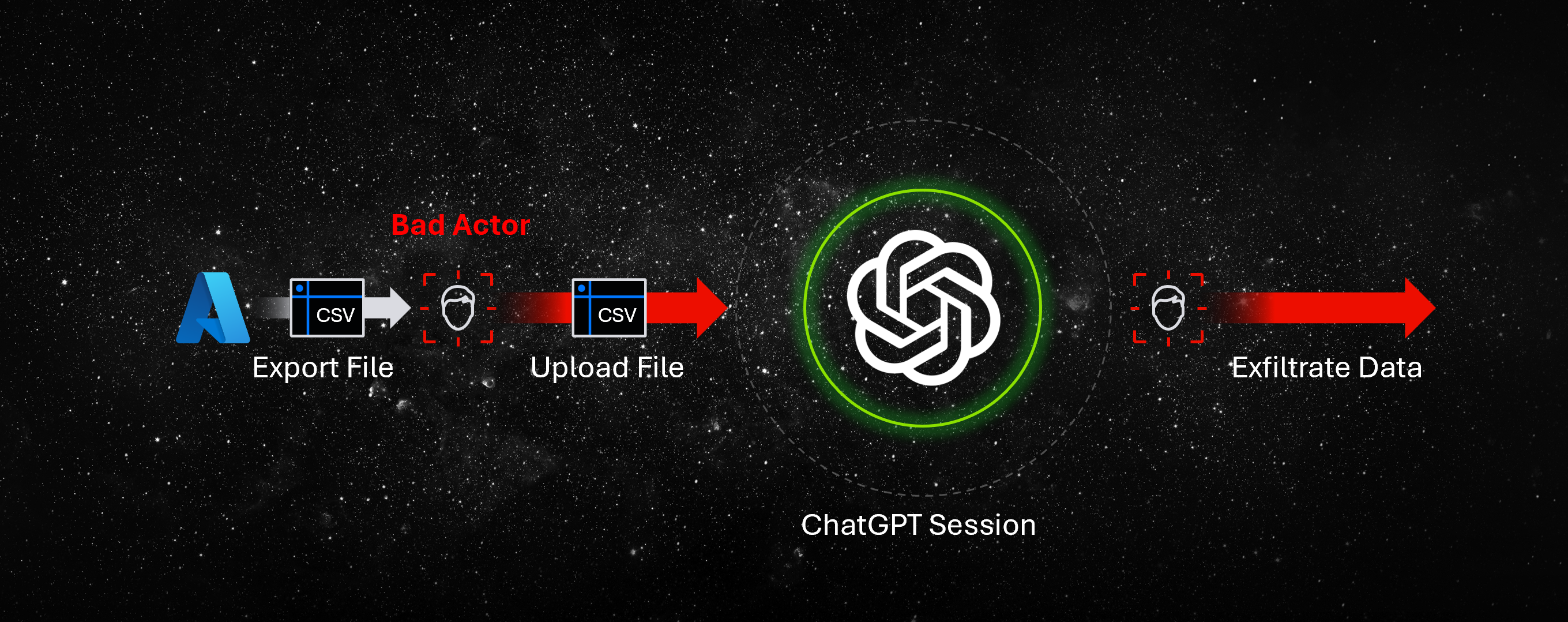
Even trusted data flows can become exfiltration paths if users are compromised.
The previously mentioned Gartner study predicts that DLP programs that can automatically and adaptively remediate policies based on real-time user risk will reduce insider threats by one-third by 2027.
Adaptive DLP for existing flows
Attackers take advantage of changes to data, the environment, and the organization. They exploit the chaos, find sensitive data to target, and slip out a newly created back door you didn’t know about. DLP solutions need to adapt to changes continuously rather than being refined manually through static list management and policy editing.
Organizational change scenario: Mergers and acquisition can add new users, new data, new environments, and new data flows. As all the ‘new’ is added, a zero-trust DLP strategy surveys all of it and assumes it is compromised. This does not mean security teams should block all actions and access. However, it does mean all flows should be monitored for risky flows of sensitive data even if they’re trusted.
A zero-trust approach also assumes that all previous DLP solutions from the acquired company may have holes. It’s your job to find them and assume they’re there.
New feature scenario: It is crazy to think about, but there was a time (circa 2018) when you could not record Teams or Zoom meetings. Transmitting certain information in Zoom or Teams chat may have been appropriate prior to the dawn of meeting recordings. After rolling out meeting/call recording, transcription, and (present day) AI agents like Copilot – your DLP policies need to adapt.
The path (a virtual meeting) remained the same, but now there are new digital artifacts (recordings, transcripts, AI generated summaries) being stored in other locations that may not be protected. Teams need to assume a new technology or functionality is compromised and adapt DLP as such.
New data type scenario: Unstructured data, such as text, images, and videos, play a crucial role in training and deploying AI models. However, securing this type of data is more complex than securing structured data. Gartner’s report emphasizes the importance of investing in specialized controls designed specifically for unstructured data to address its unique challenges.
As new data types begin to flow through existing paths, it’s imperative to deploy DLP that can flag the new type, scan, and investigate. Much like novel capabilities were created for email phishing security solutions to scan QR codes for malicious ends, DLP needs to evolve for new ways of exfiltrating data in other mediums.
Strategic recommendations for security leaders
Security leaders must prioritize DLP solutions that incorporate both behavioral and intent analytics alongside content-centric insights. With enhanced visibility into dynamic data flows, a new form of DLP can provide data lineage and a detailed map of data movement within an organization.
Zero-Trust DLP tracks where data originates, how it transforms, and where it ends up, while assuming each step in the data lifecycle is compromised. This approach helps identify potential vulnerabilities and unusual data access patterns that lead to accelerated containment and data breach prevention.
Automation will also be critical for next-generation DLP. Real-time remediation capabilities will enable organizations to respond swiftly and adapt to threats. Instead of waiting for manual intervention, an automated platform can take actions, such as isolating affected systems, blocking unauthorized access, or alerting security teams. This rapid response helps minimize the impact of data breaches and prevents further data loss.
Zero-Trust and zero-touch will be the values we hold moving forward.
What should I do now?
Below are three ways you can continue your journey to reduce data risk at your company:
Schedule a demo with us to see Varonis in action. We'll personalize the session to your org's data security needs and answer any questions.
See a sample of our Data Risk Assessment and learn the risks that could be lingering in your environment. Varonis' DRA is completely free and offers a clear path to automated remediation.
Follow us on LinkedIn, YouTube, and X (Twitter) for bite-sized insights on all things data security, including DSPM, threat detection, AI security, and more.
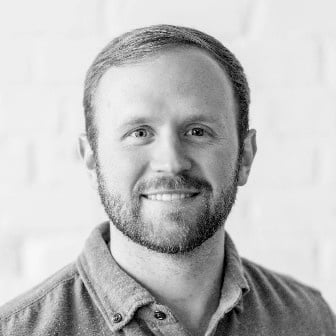